Weekend Effect in Intensive Care Units: Investigating the Impact of Admission Timing on ICU Mortality Risk
- Kaveh Sarraf
- May 26, 2024
- 6 min read
Updated: May 27, 2024
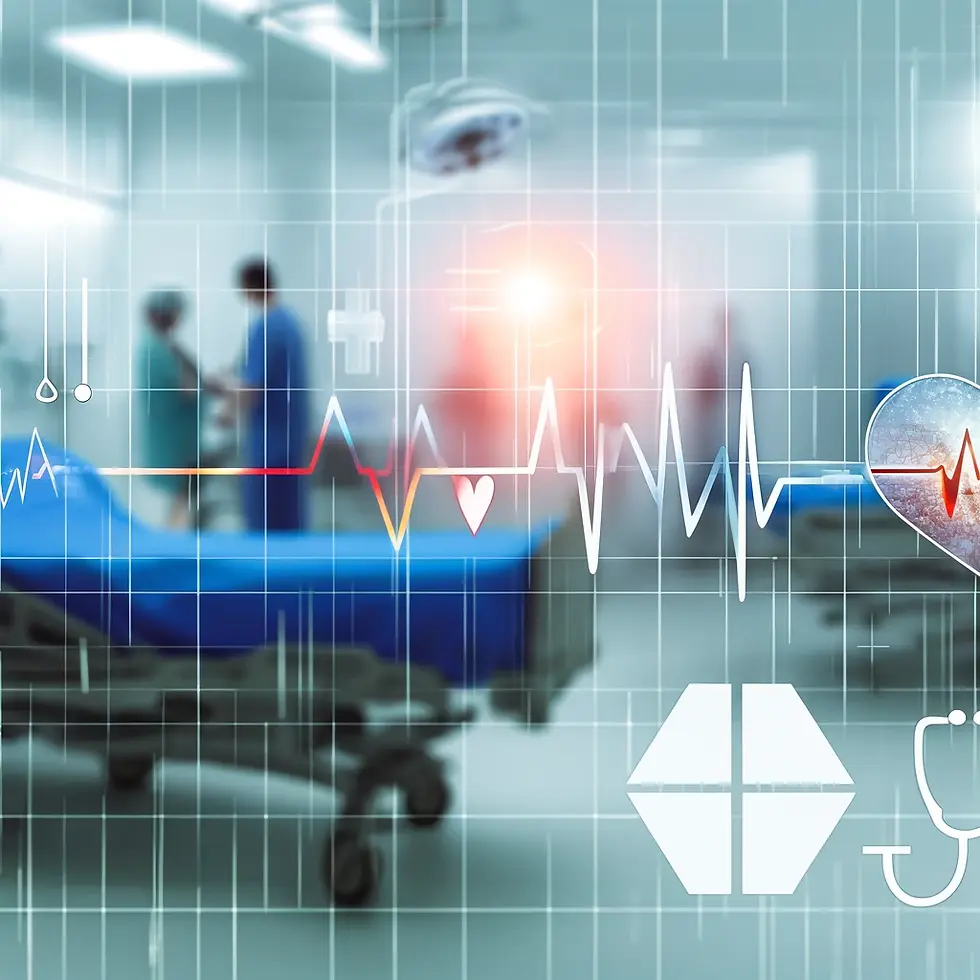
Introduction
The intensive care unit (ICU) is a critical healthcare setting that provides specialised care for critically ill patients. In recent years, research has revealed disparities in patient outcomes based on the timing of ICU admission, with some studies suggesting a "weekend effect" – an increased risk of mortality for patients admitted over the weekend.1 However, the existence and magnitude of this effect remain a topic of debate, with potential implications for healthcare resource allocation and staffing policies.
The present study aims to investigate the relationship between weekend admission to the ICU and the risk of ICU mortality. The research question under investigation is: Does admission to ICU over the weekend increase the risk of mortality? To address this question, we will develop a statistical model that estimates the effect of weekend admission on ICU mortality risk, with the ultimate objective of informing healthcare decision-making and optimising patient outcomes.
We will employ the MIMIC-III dataset, a large, publicly available dataset containing deidentified data from over 50,000 ICU patients at a single large tertiary care hospital. This rich dataset enables us to explore the potential weekend effect while controlling for relevant confounding factors, including patient demographics, severity of illness, and comorbidities. By conducting a rigorous analysis of the MIMIC-III dataset, we aim to provide a comprehensive and reliable assessment of the weekend effect in ICU and its implications for clinical practice and healthcare policy.
Method
Dataset and Pre-processing
The study population was derived from the MIMIC-III dataset, comprising de-identified data from over 50,000 ICU patients at a large tertiary care hospital. The data was pre-processed and cleaned, including removing duplicate records, handling missing values, and one-hot encoding categorical variables. The final dataset was split into training and test sets, with 80% used for model training and 20% for model evaluation.
Feature Engineering
For this study, the same feature engineering process as described in "Predicting ICU Mortality within the First 24 Hours of Admission: A Machine Learning Approach Using the MIMIC-III Dataset" was adopted. This resulted in a final dataset with nearly 500 features.
Model Selection and Feature Elimination
A logistic regression model was chosen for its suitability in handling binary outcomes. Recursive Feature Elimination (RFE) was employed to identify the most relevant covariates for predicting ICU mortality, iteratively removing the least important features based on their predictive power.
Model Fitting
The logistic regression model was fitted to the training data, incorporating 'weekend_admission' as an independent variable, along with selected relevant covariates identified during the feature engineering and selection process.
Model Evaluation
The model's performance was assessed using the test set, calculating metrics such as accuracy, precision, recall, F1-score, and the area under the ROC curve (AUC-ROC).
Effect Estimation
The effect of weekend admission on ICU mortality was estimated by calculating the odds ratio for the 'weekend_admission' variable using the logistic regression model. Odds ratios greater than 1 suggest an increased risk of ICU mortality, while odds ratios less than 1 indicate a decreased risk.
Statistical Significance
The statistical significance of the 'weekend_admission' effect on ICU mortality was determined by calculating the corresponding p-value. A p-value less than 0.05 is considered statistically significant. To estimate the standard errors for the coefficients, a bootstrapping approach with 1,000 iterations was used. Alternatively, the Wald Test was employed, assuming the maximum likelihood estimates (MLE) of the coefficients follow a normal distribution.
Model Diagnostics
Multicollinearity was assessed using the Variance Inflation Factor (VIF), with values greater than 10 indicating high multicollinearity. Model misspecification was evaluated through residual plots, with discernible patterns suggesting potential issues.
Feature Importance
The importance of each feature in the logistic regression model was analysed by examining the coefficients and their corresponding p-values. Larger coefficients indicate greater impact on the outcome, while smaller p-values suggest statistically significant relationships between the feature and the outcome.
Results
A logistic regression model was employed to investigate the association between weekend admission and ICU mortality. The model utilised a set of relevant covariates, including 'after_hours_admission', 'admission_type_EMERGENCY', 'admission_type_URGENT', among others. The model achieved an average cross-validation score of 0.9266.
Upon evaluation using the test set, the model's performance metrics were as follows:
The odds ratio for the 'weekend_admission' variable was calculated as 1.0131, indicating a slightly increased risk of ICU mortality for patients admitted on weekends. However, this effect was not found to be statistically significant, with a p-value of 0.7679.
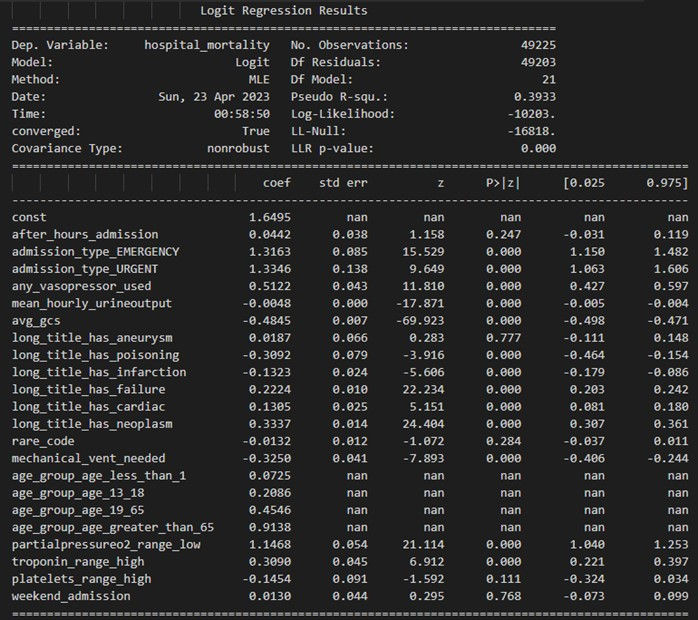
Multicollinearity was assessed using the Variance Inflation Factor (VIF), with no features exhibiting VIF values above 10, indicating multicollinearity is not a concern. Residual plots were examined to assess model misspecification, and the observed values were close to the predicted values for most data points, suggesting a well-specified model.
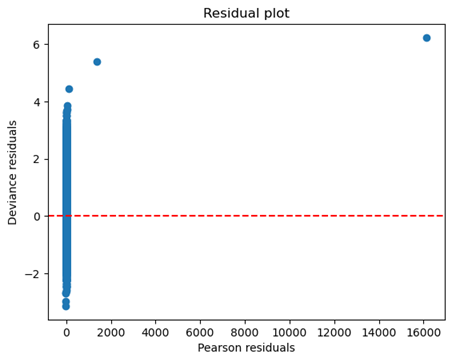
The analysis shows that the most influential predictors of ICU mortality risk are admission_type_URGENT (coefficient: 1.334561) and admission_type_EMERGENCY (coefficient: 1.316296), with positive relationships, indicating increased risk of mortality for urgent and emergency admissions. Partialpressureo2_range_low (coefficient: 1.146784) and age_group_age_greater_than_65 (coefficient: 0.913830) also have positive coefficients, suggesting higher ICU mortality risk for low partial pressure of oxygen and being in the age group greater than 65. Features such as avg_gcs (coefficient: -0.484480) and mechanical_vent_needed (coefficient: -0.325013) display negative coefficients, indicating that higher GCS scores and not requiring mechanical ventilation are associated with lower ICU mortality risk.
Several other features are statistically significant, including any_vasopressor_used, age_group_age_19_65, long_title_has_neoplasm, long_title_has_poisoning, troponin_range_high, long_title_has_failure, age_group_age_13_18, and platelets_range_high, although their coefficients are smaller compared to the aforementioned predictors.
Notably, weekend_admission (coefficient: 0.012957) has a small, positive coefficient but is not statistically significant (p-value: 0.7678752). This finding suggests that weekend admission to the ICU is not a substantial predictor of mortality risk and supports the conclusion that the weekend effect may not be present in this dataset.
In conclusion, the analysis highlights several features that significantly influence ICU mortality risk, but weekend admission does not appear to be among the most important predictors. The results provide evidence against the presence of a weekend effect in ICU admissions, although further research is necessary to confirm these findings.
Discussion
This study aimed to investigate whether admission to the ICU over the weekend is associated with an increased risk of mortality. Using a logistic regression model, the analysis provided evidence that weekend admission to the ICU is not a significant predictor of mortality. This finding suggests that the risk of mortality for ICU patients does not necessarily increase for those admitted over the weekend, in contrast to some earlier studies that have reported the "weekend effect" in healthcare.
The feature importance analysis revealed that other factors, such as admission type (urgent or emergency), low partial pressure of oxygen, and age group greater than 65, were more influential in predicting ICU mortality risk. These findings may inform healthcare providers and policymakers about the factors that contribute most to ICU mortality risk and help guide resource allocation and targeted interventions to improve patient outcomes.
The strengths of this study include the use of a large, diverse dataset with multiple predictor variables, which allowed for a more comprehensive examination of the potential relationship between weekend ICU admissions and mortality. Additionally, the application of logistic regression, a widely recognised and robust technique, enabled the estimation of the odds of mortality while controlling for various confounding factors. The study also employed rigorous diagnostic checks, such as testing for multicollinearity and model misspecification, ensuring the reliability of the results.
However, there are several limitations to consider. First, the study is based on observational data, which inherently limits the ability to infer causality. Despite adjusting for multiple potential confounders, there may still be unmeasured variables that influence the relationship between weekend ICU admissions and mortality. Second, the dataset used in the study is subject to potential inaccuracies and missing data, which could affect the validity of the findings. Third, the generalisability of the results may be limited due to potential differences in healthcare systems, patient populations, and ICU settings across various regions and countries.
Future research could address these limitations by conducting more extensive analyses using datasets from different healthcare systems and patient populations to enhance the generalisability of the findings. Additionally, researchers could explore other analytical methods, such as propensity score matching or instrumental variable analysis, to further address potential confounding and strengthen causal inferences. Investigating the impact of weekend ICU admissions on patient outcomes beyond mortality, such as the length of ICU stay or readmission rates, could also provide valuable insights into the quality of care delivered during weekends. Finally, examining potential moderating factors, such as staffing levels or ICU resources, may help identify specific areas for improvement to ensure optimal patient care throughout the week.
Conclusion
In conclusion, this study found no evidence to support the notion that weekend admission to the ICU is associated with increased mortality risk. However, due to the limitations outlined, further research is warranted to confirm these findings and to continue investigating the factors that contribute to patient outcomes in the ICU.
References
Bray, B. D., & Steventon, A. (2017). What have we learnt after 15 years of research into the 'weekend effect'? BMJ Quality & Safety, 26, 607-610. doi: 10.1136
You can access the code used for this predictive model on GitHub by clicking on the link below:
Comments